How airlines' cargo business can earn better yields by applying AI, ML
India currently has 3 mn tonnes of air cargo annual throughput and the gov has set up a target of 10 million by 2030.
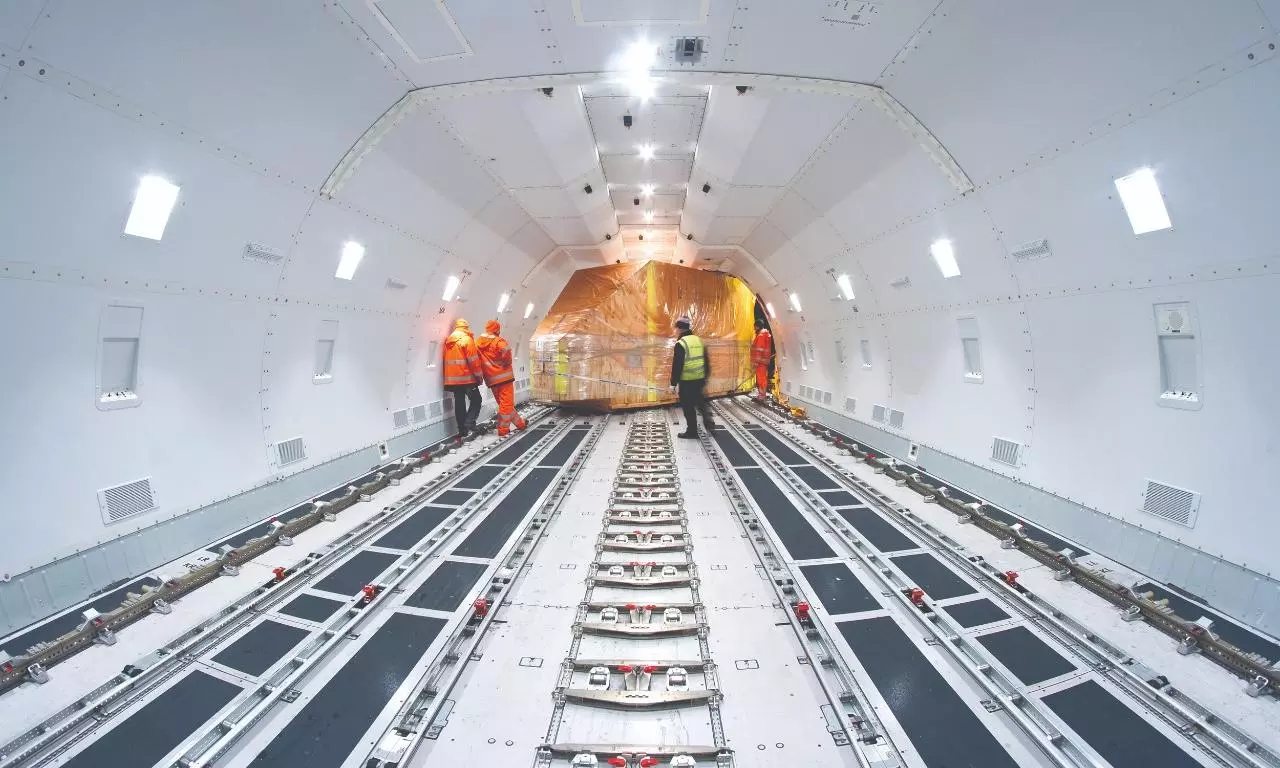
"A well thought-through data-driven analytics strategy can bring better yields, become more responsive, improve efficiencies and deliver better customer satisfaction.": Ashok Rajan, IBS Software
As a capital intensive industry, air cargo needs to innovate SOPs and optimise operations to stay in the business. It is particularly important when the market is moving through a rough and volatile period in history. Thus any technology that can reduce the complexity and cost would see a high adoption rate. AI, ML and data visualisation have already proved not just in managing the cargo business for airlines but also in improving their yields.
In the recently concluded annual event of Air Cargo Forum India (ACFI), union minister of civil aviation Jyotiraditya Scindia emphasised that the growth potential for air cargo in India is tremendous and he is certain that India will emerge as a global air cargo player in the coming years.
India currently has close to 3.1 million tonnes of air cargo annual throughput and the government has set up an ambitious target of 10 million by 2030.
"We will develop a hub and spoke arrangement and build transhipment hubs across India. Giving a boost to the agri sector, we are targeting 53 cargo terminals connecting airports with agriculture farmlands for the transport of perishable commodities. So now, green shoots from Assam or Tripura's jackfruits can travel to London and Germany rather than only being consumed internally in Kolkata, Hyderabad, Chennai, etc," he said.
Indeed, cargo has proven itself to be a key revenue generator for Indian airlines in the chaos of the global pandemic, and now the stage is set for air cargo to grow into India's globalized future. However, the industry is plagued with many inefficiencies and challenges, which would keep itself away from the potential it has. One way to solve this is by looking into technological solutions.
"High dwell times, congested cargo terminals, sub-optimal use of belly cargo capacity, missing/damaged non-traceable cargo, and manual processing are potential for AI and ML in the immediate future. Autonomous trucks/aircraft and AI-powered robots are a few of the long-term future in India," says Sabari Ramnath, industry expert of freight logistics solutions, Unisys.
He has no doubt that it will transform the Indian air cargo industry, however, he warns that the future depends on how our business leaders look through the AI/ML lenses to identify new business models and new revenue sources and bring in critical efficiencies.
Amar More, chief executive officer, Kale Logistics Solutions, perceives technology as the key that will unlock the Indian air cargo industry's growth rate.
"With the digital impetus coming from the government like Gati Shakti, National Air Cargo Policy, airport digitization, and cargo modernization, technologies like AI, BL, blockchain, data will form the augmented ring for this industry," he said.
Ashok Rajan, senior vice president head – cargo logistics business, IBS Software, reports that these technologies are evolving at an extremely fast pace and the immediate trend that he can see is the "democratization" of such technologies.
"Traditionally, such cutting edge capabilities were only accessible to large businesses with massive resources. This scenario is quickly changing and will continue to change in the coming years, making these tools more and more accessible to any size of business," he said.
The business of air cargo demands investments in aircraft, equipment, or other fixed assets which represent a sizable expense. Thus airlines need to optimize their operations to reduce costly inefficiencies. Artificial intelligence (AI) and machine learning (ML) can enable air cargo to increase visibility, make improvements, be more efficient and do informed decision-making.
High dwell times, congested terminals, sub-optimal use of belly capacity, missing/damaged non-traceable cargo, and manual processing are potential for AI and ML.
Sabari Ramnath, Unisys
Why should airlines invest more in AI and ML?
Among the varied uses of AI, More notes that it has already proven to be useful, "especially in making predictions."
He said that by analysing historic flight information, managers have been able to optimally plan their operations. "In a volatile context where the available staff and equipment are restrained, smart algorithms enhance resource allocation based on requirements," he added.
He pointed out that the reduction of Co2 emissions is another benefit which airlines can derive from AI, ML and big data.
"By combining data points from planes' communication systems with real-time data about the flight itself (such as weather and loads), these technologies are able to determine how efficiently fuel is being used during any given flight. This information is then communicated to pilots, along with suggestions for actionable changes, such as reducing acceleration altitude," he said.
Air cargo is in the business of selling cargo capacity, a highly complex and dynamic asset. How the airlines identify, package and sell this asset to the right buyer at the right time and market conditions determines the maximum yield they draw from the asset. Cargo may take only hours to travel from origin to destination but may stay on the ground for days before reaching the consignee.
According to Rajan, AI, ML and analytics are the capabilities that will save almost any industry from commoditization in the future, not just airlines.
He notes that air cargo provides some unique challenges for traditional statistical functions like forecasting and reports that they have been able to see improvement in accuracy of results by adopting AI and ML based algorithms for these functions.
"Intelligent automation in solutions that sell air cargo can transform the airlines' cargo business like never before. Above competitive advantage, a well thought-through and implemented data-driven analytics strategy can bring better yields, become more responsive, improve efficiencies and deliver better customer satisfaction levels that encourage repeat business," he said.
What does AI/ML on ground mean?
AI is a broader term that describes applications that understand, think, learn, and behave like humans. Whereas ML is a subset of AI, it allows the machines to learn and make predictions based on their experience (data).
Ramnath identifies two use cases: customer service and dynamic pricing. AI-powered virtual assistants (or chatbots) allow airlines to better engage with customers and in the case of pricing, "airlines use algorithms to forecast demand and set prices for years."
"But, using AI/ML, air cargo can dynamically recommend products and set prices that appeal to individual consumers," he adds.
According to Rajan, the most common and widespread area for analytics based algorithms and AI in air cargo is in revenue management and more specifically in the area of dynamic pricing.
"As the industry adopts digitalization in the sales process, pricing and offer management need to become smarter and ubiquitous – in contrast to the reactive business process prevalent today," he says.
Rajan also noted that AI and ML have many more applications such as in planning, warehouse automation, resource management and automation of business processes. He also predicts that as the cost barrier to such technology diminishes due to advancements in computing, the industry will see more such cases becoming part of day-to-day use.
Kale Logistics Solutions has been a pioneer in cargo community systems and has integrated AI, ML and data visualisation to transform the air cargo operations. They have used AI in geofencing for truck slot management at the airport complex.
More says, "Transporters can dock-in with the mobile app when in the premises on the airport. This saves significant time for both the transporter and the cargo handler to handle cargo."
Kale Logistics Solutions uses ML in converting PDF, scanned documents or emails to electronic Air Waybills (e-AWB). Their tool – PING reads the data from these sources and picks the relevant data fields to create the standard e-AWB and file with the airline. "This can be done in less than 3 minutes," he adds.
Data visualisation is another crucial area which is gaining a lot of ground, data around top customers, products, seasonality, processing times, revenue leakages and equipment utilisation for an airport or handler can lead to better customer service, profitability and process efficiency.
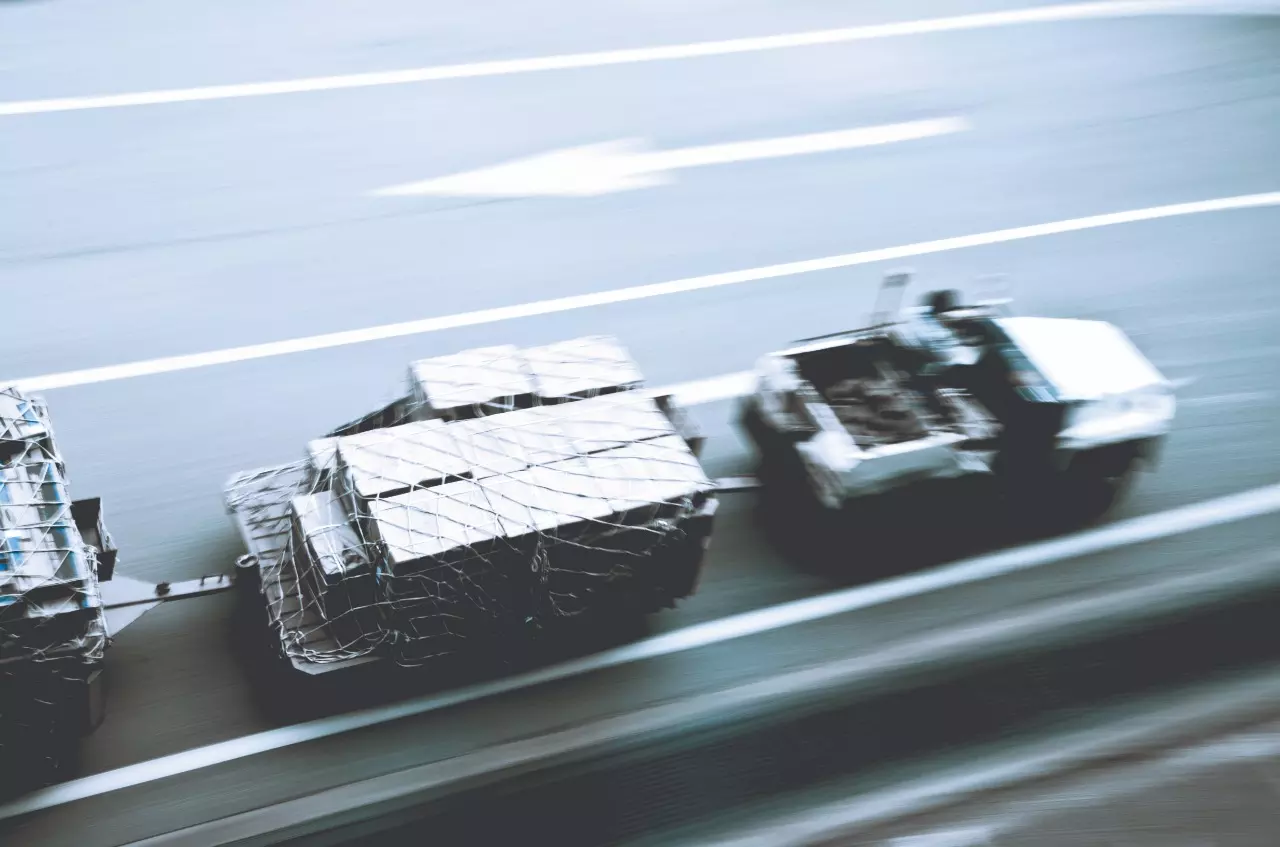
How about data visualisation
More notes that we are in the era of predictive commerce.
He says that it's time for air cargo to help the customers to find products in the precise moment of need, "perhaps before they even perceive that need, " as he puts it.
"The ML algorithms would understand human behaviour with large-scale automation and data integration, and predict exactly what customer needs, " he adds.
For Ramnath, it is not just about building dashboards with lots of charts and filters. Instead, data visualisation means representing insights from the data.
And for him, data visualisation could be one or a mixture of these two types of dashboards. "Operational dashboards display real-time metrics. E.g., a dashboard to display the performance of the shipments and alert operational teams to anomalies. Strategic dashboards present key performance metrics to senior management and aim to tell the 'big-picture' story behind the data. E.g., a sales dashboard to display the cargo sales insights to the cargo sales heads or head of cargo to make the decision, " he says.
The algorithms would seem cool but in the absence of the right and quality data, it is impossible to achieve those results.
Collecting the right data
We cannot build an AI strategy if we don't have the right data. The AI/ML models are built and learned from data. The first step of creating a model is data collection or data preparation — extracting inputs to the model from data. So, if the data is incorrect, the entire model is incorrect. Hence, ensuring the quality of the data is paramount.
Ramnath explains that there are four components which will ensure data quality – called CATCI (Completeness, Accuracy, Timeliness, Consistency, and Integrity).
He says, "Completeness ensures there are no gaps in the data between what was supposed to be collected and what was collected. Accuracy ensures the data collected is correct, relevant, and accurate. Timeliness ensures the data is received at the expected time for the information to be utilised efficiently. Consistency: ensures the data is aligned or uninformed with another dataset. Integrity ensures that all data can be traced and connected to other data. "
As an industry, air cargo is very disparate in technology adoption and is not connected to the entire cargo community, according to More. So, data is re-entered 14 times in the value chain manually leading to duplication, errors and incomplete data.
"Cargo Community Systems (CCS) essentially form a network on all the logistics stakeholders (shipper, consignee, transporter, freight forwarder, customs, cargo handler, airport, airlines) who are connected on a common portal to exchange all trade related data which is useful the next in line stakeholder. The CCS also checks for data discrepancy with its in-built intelligence and prompts the users to correct any incorrect data. This thereby ensures data accuracy, authenticity, speed of operations and transparency of trade, " he said.
Meanwhile, Rajan notes that there are three key steps towards developing a data-driven and analytics strategy for any business.
As he puts it, "One, you need to pin down the outcomes that you are driving towards, which should then define your data needs. This is more complex than it looks as good data is the most critical resource in any analytics project. The second step is to evolve business practices around analytics and start thinking about how such services are going to be consumed by your business. Thirdly, you need to start thinking about the right platforms and IT environment that can transform the business data into insights that help to make decisions to eliminate the "Chinese Whisper" problem and ensure that all stakeholders are consuming the same data. "
Indeed, there is room for improvement and technologies like AI ML and data visualisations can solve them. However, more than anything we would need good leaders with the right vision to help use technology for the right reason.
This article was originally published in Indian Transport Logistics News' May - June 2022 issue.
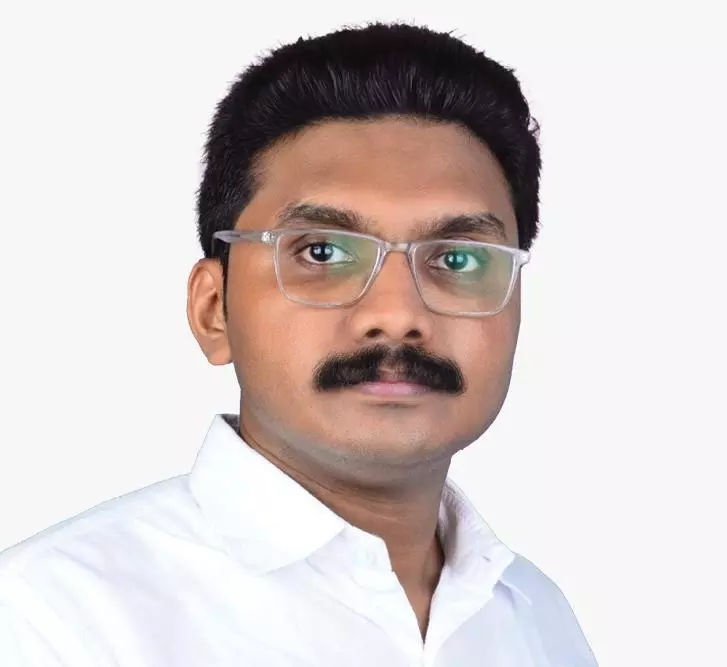
Libin Chacko Kurian
Assistant Editor at STAT Media Group, he has six years of experience in business journalism covering food & beverage, nutraceuticals and now logistics. His current passion is to understand the nuances of global supply chains and their current turmoil. Outside work, he is also interested in philosophy, history, birding and travelling. Mail him: libin@statmediagroup.com Follow on LinkedIn